Read the White Paper: Clinical Analytics: The Journey from Problem Identification to Solution
Encouraged by Health Authorities, Life Sciences organizations have moved towards risk-based approaches in almost all of their activities. To support a true risk-based approach in clinical trials, information from a variety of sources must be unified and analyzed to present an accurate understanding of status, risk and progress.
For example, risk-based clinical quality may require information from not only the Clinical Trial Management System) CTMS and Electronic Trial Master File (eTMF), but also Electronic Data Capture (EDC), Electronic Patient Reported Outcomes (ePRO), Randomization and Trial Supply (RTS), Regulatory Information Management System (RIMS) and Quality Management System (QMS). Without automation, the process of populating and analyzing data is both resource-intensive and error-prone.
Looking for more details? Read our White Paper on this topic.
Dashboards and analytics should enable users to reach conclusions and assess priorities easily. But there’s a lot to think about before you can create analytics that truly aid in identifying risk, understanding status, uncovering and addressing inefficiencies and bottlenecks, and prioritizing work.
Understanding the Purpose of Metrics
Analytics can be grouped into four major categories:
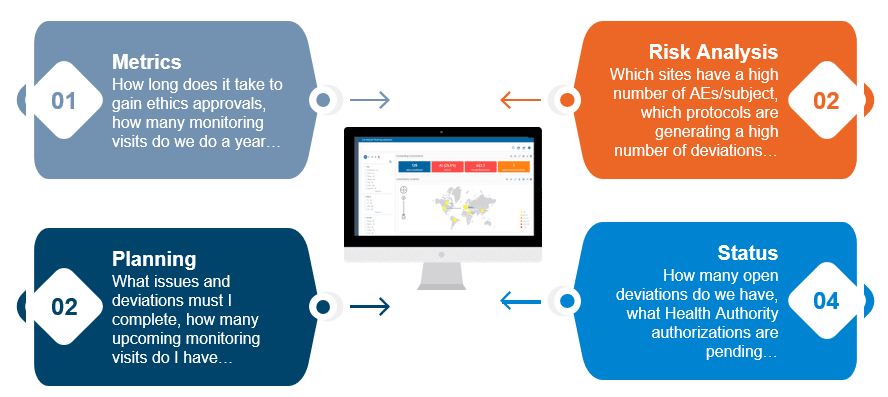
- Metrics. These are usually Key Performance Indicators (KPIs) which are often reported periodically to upper management and often used, rightly or wrongly, as a measure of an organization’s success. Metrics can often be thought of as information for the 1%.
- Risk analysis. These analytics report against predefined risk factors, that is, the potential for adverse consequences. They include information on outliers and anomalies as well as out of specification conditions. They are often used by those responsible for managing processes or business units.
- Planning. These analytics report on or estimate upcoming workload, by using awareness of known tasks and due dates, by projecting work based on known factors, or by using Artificial Intelligence algorithms to predict. These are truly analytics for the 99% since at some level they provide useful information to almost everyone in an organization.
- Status. These analytics provide a snapshot of where an organization stands at a point in time by reporting on finished and unfinished business.
Within this framework, an organization can identify specific sets of metrics that will support reaching organizational objectives. These metrics should answer the questions about what each associate needs to know in order to plan, manage or mitigate processes and risks in clinical trials. When identifying what metrics to analyze, considerable insight can be gained by studying TransCelerate’s Risk Indicator Library.
Defining and Presenting Data
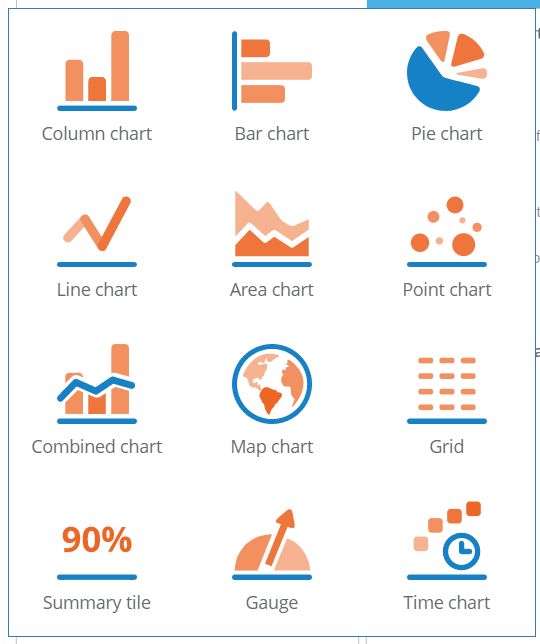
After desired metrics are defined, the next step is to determine whether it is really feasible to collect them, and at what cost in terms of time and effort. Once the feasibility of collecting is determined and any necessary cost-benefit analysis is completed, the presentation of metrics in analysis dashboards can be defined.
Dashboards with well-chosen visualizations help users to identify and manage risk. Choosing the right data visualization is important. Data visualizations should be selected and organized into dashboards that trace back to the key goals of identifying risk, understanding status, planning work, and increasing efficiency.
Summary
Defining a set of useful metrics to support planning, monitoring and managing clinical studies using analytics requires making a set of sound decisions, not just using what a vendor offers. Best practices include:
- Understanding what you want to measure – consulting industry sources for ideas as well as working with the user community.
- Defining metrics that support risk management, operational efficiency, status reporting, and workload management.
- Ensuring that the purpose, use and audience for each is well-defined.
- Thinking about whether data can truly be used to compare across studies, sites or associates.
- Defining how meaningful data can be collected, and consider cost-benefit in choosing metrics.
Get free access to the White Paper: Clinical Analytics: The Journey from Problem Identification to Solution